How can lenders optimise predictive analytics and machine learning to make the most reliable decisions? Get it right and lenders can reduce default rates and decrease the burden of debt for consumers across the UK, argue Campbell Scott and Hitendra Patel
Although the Financial Conduct Authority (FCA) in the UK requires lenders to assess consumers’ ability to absorb additional debt when making credit decisions, unsecured debt has been rising rapidly across the country since the 2008 financial crisis.
Currently, more than 3 million UK credit card holders are trapped in a state of persistent debt, where the repayments made on a monthly basis are less than the interest and changes on the account.
While this specific issue has been addressed by the FCA, of greater concern are those consumers who, on the face of it, seem to be coping with their current financial burden, but who are under a substantial level of pressure to meet their commitments, which is not reflected in a typical credit score.
Poor lending decisions lead to higher default rates and impair consumers’ lives. How, therefore, can lenders in the UK better identify the consumers who cannot afford to repay their loans, or where the offering or extension of credit will have significant detrimental effect on the lives of that consumer?
How well do you really know your competitors?
Access the most comprehensive Company Profiles on the market, powered by GlobalData. Save hours of research. Gain competitive edge.
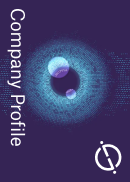
Thank you!
Your download email will arrive shortly
Not ready to buy yet? Download a free sample
We are confident about the unique quality of our Company Profiles. However, we want you to make the most beneficial decision for your business, so we offer a free sample that you can download by submitting the below form
By GlobalDataLenders evaluate credit risk based on credit scores, which measure the likelihood that a credit agreement will be repaid. Credit risk models take the data from the application, or the behaviour of the account or customer, to predict payment performance.
However, there is growing awareness that credit risk scores alone do not give a full enough picture to accurately determine the risk of default.
Industry regulators are putting increased scrutiny on ‘affordability risk’ – whether credit commitments can be repaid, or whether taking on additional debt on one trade line may even cause other trade lines to default – as a criterion for measuring consumer creditworthiness, reinforcing the notion that credit risk assessments need to be made more robust.
The affordability gap
The risk of non-repayment is determined by two traits: ability and willingness. The former is the same as affordability – the ability to repay the outstanding lending – and the latter refers to the consumer’s commitment to repay.
Credit bureau scores can only predict the overall odds of repayment for the consumer. They can’t assess which of the two traits is driving non-payment in a particular case. In order to separate out and quantify affordability across the life of a credit obligation, new tools are required.
In addition, it is sometimes necessary to understand how balance increase will impact a consumer’s probability of default – another situation that cannot be assessed with a credit score alone.
Credit risk metrics need to be supplemented by affordability risk measurement tools, as consumers with the same level of credit risk – the same credit risk score – can have different repayment outcomes depending on their affordability risk, if they substantially increase their balances.
Casual analytics
To gauge affordability, lenders need both simple metrics about the consumer’s current situation – for example, whether they have been making only minimum payments on a credit card – as well as a more complex overview of the overall consumer profile.
This is where analytics are required – to first enable the selection and organisation of the most crucial data points and then to express these outputs as easy-to-use metrics.
In our research, we created a Balance Change Sensitivity Index (BCSI) – an analytic affordability model that was developed to rank-order individuals according to their sensitivity to a sizeable future increase in total credit card balances.
This analytic can help plug the gap of balance sensitivity, a factor that is inadequately considered in current risk metrics. Unlike credit scores, which use associative or correlational models, BCSI relies on a causal modelling approach – if an individual is balance-sensitive and given a sizeable increase in their balance, this is more likely to cause a subsequent increase in default.
Our research finds that credit risk is somewhat positively associated with affordability risk, however, the correlation is only mild. In each risk score band, we find consumers with low and high affordability risks. This debunks the common assumption that high credit risk equals lack of affordability or vice versa.
With the additional insight from BCSI, lenders can refine their card limit strategies to extend less credit – or even reduce limits – to balance-sensitive customers and provide more products and obtain an increased share of wallet from the more stable customers. Alternatively, the same model could be used to assess a consumer’s ability to pay back a higher minimum repayment, which may be required under the FCA requirements on persistent debt, to understand whether the consumer would need to be treated with forbearance.
Ultimately, BCSI will help identify opportunities to improve the quality of the portfolio, both from the perspective of profitability and from a compliance perspective, treating customers in a manner that does not adversely impact their quality of life.
Finding a solution to the affordability gap
Although lenders can ask consumers for evidence of their income and expenditure during the origination process, it is unfeasible to continually request updated paperwork to determine affordability, and a consumer’s cash flow and affordability do change over time. This makes it difficult for lenders to decide who can afford an increased credit line, or would be ideal to cross-sell new credit products to.
In order to help UK lenders mitigate affordability risk across each consumer’s lifecycle and address compliance requirements, FICO joined forces with Equifax to create the FICO Risk and Affordability Decision Suite (RADS). This uses advanced predictive analytics and machine learning to develop nearly 50 decision keys – including the BCSI and a new customer management risk score – to assess both a consumer’s repayment risk and their ability to handle existing and new credit.
In addition, RADS delivers up-to-date data directly from Equifax to a lender’s decision engine. This enables lenders to make the most reliable decisions to drive reliable, profitable growth in a seamless end-to-end process, without the need for significant IT resources
The credit worthiness of any individual lies at the core of all lending decisions. Affordability risk is the new dimension on the list of requirements for responsible risk management.
The next generation of market-ready credit solutions need to combine data with innovative analytics to help lenders remain compliant and identify both credit opportunities and restrictions.
By considering both credit and affordability risk, lenders can create tailored solutions for each consumer that are fit for purpose, reduce default rates and decrease the burden of debt for consumers across the UK.
Read more about affordability analytics at www.fico.com/en/products/fico-risk-and-affordability-decision-suite-equifax