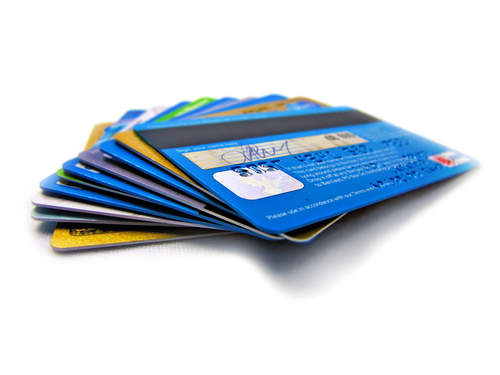
Which works better for modelling credit risk: traditional scorecards or artificial intelligence and machine learning? Given the hype surrounding AI today, this question is inevitable, but it isn’t an either-or conundrum. The best approach is to figure out how to use them together. FICO’s Scott Zoldi writes
Exploring analytic “mash-ups”
The reality is that traditional scorecard methods and AI bring different strengths to credit risk modelling. In traditional risk modelling, customer segmentation is based on ‘hard’ lines and broad categories, such as new customer vs. existing customer.
In contrast, you can use AI and machine learning algorithms to discover a better way to segment the scorecards. This allows you to apply AI to improving risk prediction without creating ‘black box’ models that don’t give risk managers, customers and regulators the required insights into why individuals score the way they do.
Our data scientists are now starting to use techniques such as collaborative profiling to reveal entity segmentation based on customer behaviours. We can then group customers into micro-segments based on their shared behaviours, instead of typical segmentation approaches that rely on hard business attributes.
Incorporating these subtle behaviours into credit risk modelling provides the best of both worlds for customers. It uses the traditional, time-tested analytic models and scorecards, but enhances them with AI to drive better segments and feature creation in models, resulting in more predictive scorecard models.
How well do you really know your competitors?
Access the most comprehensive Company Profiles on the market, powered by GlobalData. Save hours of research. Gain competitive edge.
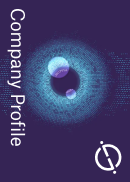
Thank you!
Your download email will arrive shortly
Not ready to buy yet? Download a free sample
We are confident about the unique quality of our Company Profiles. However, we want you to make the most beneficial decision for your business, so we offer a free sample that you can download by submitting the below form
By GlobalDataAnother approach is to use AI and machine learning to build new types of credit risk models to capture maximum predictive power, and find new relationships amongst input features which can produce a stronger model. For example, utilisation is always an important feature in a credit model, as is delinquency, but a nonlinear combination of these can produce more optimal results in a machine learning model.
These new inputs can then be driven into a traditional scorecard model to ensure they are easily explainable. The same techniques are essential when considering non-traditional data sources where the most predictive combinations of inputs are yet to be discovered.
Improving results
The following examples illustrate how you can achieve better performance and clarity by combining the machine learning and scorecard-based approaches.
When developing a credit card churn model, FICO data scientists used machine learning to identify a powerful interaction between the frequency of card usage, and how recently it has been used. Including this interaction as a nonlinear input feature in an interpretable scorecard led to a substantial improvement of approximately 10% in predicting credit card attrition.
In addition, another 15% improvement in performance was found by applying machine learning with a much larger set of features relating to event-specific recent occurrences and frequencies. These predictive improvements in turn can translate into substantial portfolio profit gains and a much more precisely targeted retention strategy.
In a project to build home equity risk models with limited data, the lack of enough poor performing loans in our sample was causing lower performant traditional scorecard models. By building a machine learning model, we were able to confirm that we were losing a significant amount of signal by relying on a traditional scorecard which required great amounts of bad coverage.
Machine learning led us to change the model performance outcome from a binary outcome to a continuous outcome. By combining this machine learning model insight with scorecard technology, we created a strong, robust solution and saw a 20% improvement in model performance over the traditional scorecard model alone.
A smarter way to use AI
As a data scientist who has spent his career developing business-ready AI applications — I have 38 granted patents in this space (and 41 pending patent applications) — I understand the power of AI and machine learning. Today, however, I see a lot of naivety regarding how it can be used.
You don’t want to throw in a lot of new data sources – many of which may not be permissible in credit decision-making, and might be easily manipulated (like social media data) – into an AI model that comes up with a score that may not be explainable. Lenders in many markets need to be able to explain how a customer was scored, and it’s important regardless of regulators to understand what relationships are being learned from this data, and if these relationships really matter to the business.
It’s unquestionable that AI presents a host of exciting opportunities for the finance industry. However, above all, it’s important to ensure they are managed in a way that produces reliable, explainable results that drive better outcomes for customers.