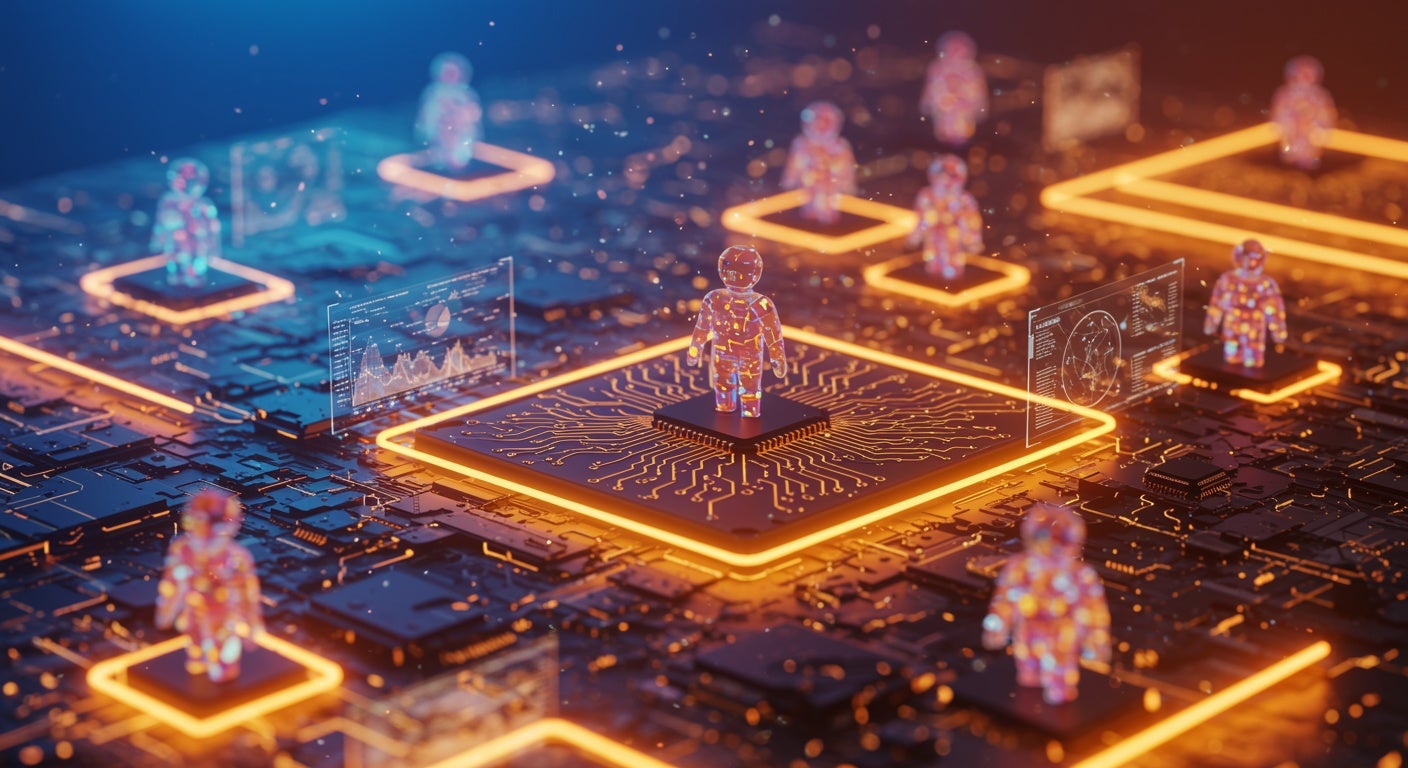
Agentic AI is the next step change in enterprise AI technology. And while, in theory, it presents limitless opportunities for increased business efficiency and growth, the risks need to be approached with intention.
“As enterprises begin to adopt agentic AI, they’re shifting from prompt-based assistants to intelligent systems that can reason, plan, and take action across tools,” says Joel Hron, chief technology officer at Thomson Reuters.
And while agentic AI can unlock enterprise workflow transformation, it also introduces new risks. “We’re now coordinating non-deterministic, self-directing agents that execute tasks on behalf of users. Without proper context management, seamless tool integration, and human-in-the-loop user experiences that provide transparency to end users, it’s a recipe for invisible failures,” says Hron.
What is agentic AI?
The primary characteristic of an agentic AI system is its ability to make decisions and perform tasks autonomously, though most systems still have a human-in-the-loop.
The autonomy of agentic AI systems differentiates them from GenAI systems like OpenAI’s ChatGPT which work, instead, on a request response basis. GenAI tools like ChatGPT have seen massive adoption and the trajectory towards more autonomous tools has led to agentic AI becoming the next logical step in the overarching path towards artificial general intelligence.
Agentic AI is being developed to include increased access to more tools. These may include anything from a web browser or a programming environment, or access to real-world processing tools such as credit cards. As the number of tools at agentic AI systems’ disposal grows, so too will the complexity of tasks it can address.
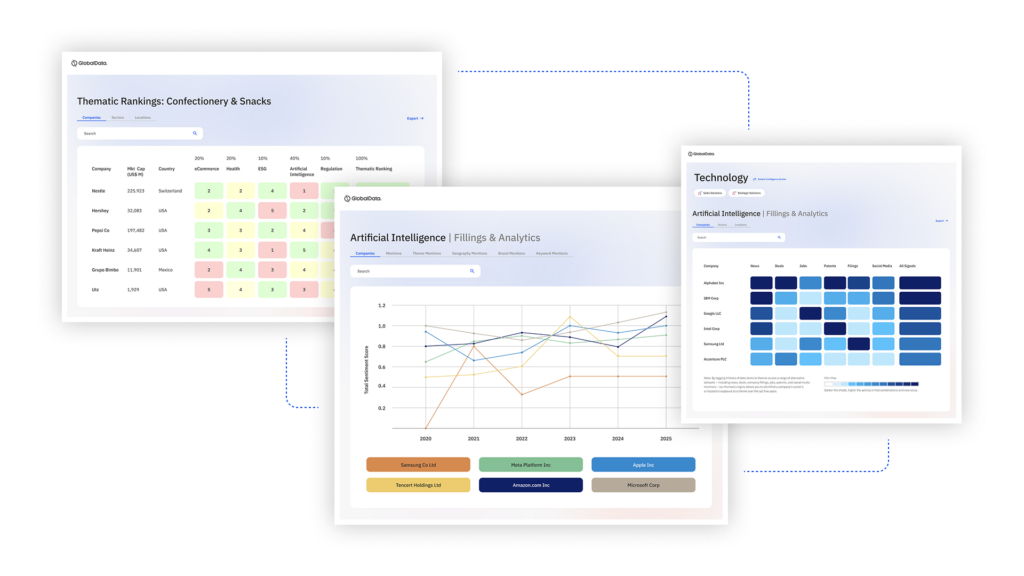
US Tariffs are shifting - will you react or anticipate?
Don’t let policy changes catch you off guard. Stay proactive with real-time data and expert analysis.
By GlobalDataAnother significant characteristic of agentic AI systems is that while a request response style tool stops working when it delivers response, agentic AI agent will continue working until it determines that it has finished executing a task. Otherwise, it will refer back to the human-in-the-loop in the case of an error or when it requires more information to complete the task.
At present, agentic AI can run a task anywhere from 30 seconds to half an hour. As the run time capacity increases, agentic AI will have the ability to carry out increasing amounts of human labour.
The risks of rushing to implementation
At Thomson Reuters, Hron has trained its agents to reason like professionals and use the company’s products in the same way professionals do by executing safely within established best practices.
“Our success hinges on this alignment between agent autonomy and domain expertise. The technology is ready — but adoption must be guided by standards, transparency, and user experiences that reinforce trust throughout the workflow,” he says.
Andrea Carnelli Dompe is co-founder and CEO of private markets AI company Tamarix, which builds agentic AI tools that act as a co-pilot for private market investors—a business for which trust is key.
Dompe’s clients are often “drowning in unstructured data and repetitive workflows” and struggling to extract investment data from PDFs, emails, and portals. “We solve this by deploying supervised agents that automate document retrieval, document review, data entry, and portfolio monitoring—freeing up time and improving accuracy across the board,” explains Dompe.
The impact on workflow transformation is massive. Dompe’s clients report over 10x productivity gains and reduced error rates.
But the biggest risk, according to Dompe, is the gap between expected and actual performance—especially when agents are left unsupervised on mission-critical tasks requiring 100% accuracy.
“We mitigate this by setting clear benchmarks, flagging uncertain outputs for review, and making it easy for users to correct and train the system. This continuous feedback loop ensures agents improve over time, allowing private markets investors to scale intelligently without compromising trust,” says Dompe.
Tamarix offers “self-serve” model where customers interact with agents directly, and a “fully managed” option where Tamarix supervises the agents to guarantee outcome quality. Dompe says that this flexibility lets clients choose the level of oversight they need—whether for internal ops or external reporting.
First steps to agentic AI integration
The determining factor for the effectiveness of any AI agent is ultimately the quality and governance of the data that powers it. And with any implementation, addressing legacy systems integration needs to be prioritised over a rush to implementation.
Sumeet Agrawal, vice president of product management for agentic AI at Informatica, notes that we’re just at the start of an agentic AI wave of innovation that could play out for years. And while there is much enthusiasm about its potential benefits, agentic AI is still delivering limited value creation. “We need to move from experimentation to tangible ROI (return on investment),” says Agrawal.
Agrawal returns to the question of underlying data. “Before diving into agentic AI, companies must lay a solid foundation by prioritising high-quality, well-governed, and accessible data. Without this, even the most advanced AI will struggle to deliver. Businesses should start by investing in strong data governance, workforce training, and curated, reliable datasets. Those who get the fundamentals right will be best placed to unlock the full potential of agentic AI at scale,” he says.
Businesses also need to decide whether to use open-source versus proprietary data based agents. This, says Agrawal, should depend on an organisation’s specific needs, resources, and priorities,
“While open-source agents offer flexibility and customisation, proprietary agents typically come with pre-built integrations, enterprise grade support, and robust security out of the box, which can save time for businesses looking for faster deployment,” he says.
Agentic AI: what could go wrong?
Chief AI architect at UST, Adnan Masood, has advised a number of global CIOs on the risks of agentic AI deployment. High-profile examples of corporations deploying too soon include an Air Canada passenger winning small claims court proceedings in February 2024 in a case in which the airline’s chatbot hallucinated an incorrect refund policy.
In June 2024, McDonald’s ceased operating its AI automated voice ordering service in more than 100 of its drive-through restaurants after it was said to be processing around 15% of orders incorrectly and required human intervention for around one-in-five orders. Furthermore, the mishaps documented on video by customers went viral online, causing considerable reputational damage to the company.
In 2023, Chinese AI company iTutor became the first company to settle a lawsuit based on employment decisions made using AI in which the US Equal Employment Opportunity Commission accused the company of using AI to eliminate older job applicants.
Masood warns that skipping data hygiene, skimping on integration, and giving AI agents unfettered permissions, will mean that, “the blast radius is wide”. Agentic AI deployment must follow a “copilot-until-proven” doctrine, says Masood. By this he means read-only access, sandbox pilots, red-team stress tests, and a human sign-off until an AI agent’s precision gap versus human analysts falls below 0.1 percentage point in blind A/B testing on live data.
“My recommended best practices are to budget for these guardrails up front and the ROI follows, 30% faster customer resolutions and eight times dev velocity are typical in our rollouts.
“Treat privacy breaches, drift, and rogue autonomy as priority defects, measure success in business KPIs like hours saved and errors averted, and scale in phases. Done this way, agentic AI moves from hype to balance-sheet impact—and the board will notice for the right reasons,” says Masood.
What’s next for agentic AI?
Multi-agent AI systems are the logical progression from agentic AI. HCL Tech offers AI agent products that detect defects and anomalies in various industrial sectors, with real-time dashboards and virtual assistance to reduce costs and improve production.
The technology is having a profound impact on the company’s enterprise clients, according to HCL Tech Alan Flower, head of AI & cloud native labs. And multi-agent agentic AI is on the horizon.
A multi-agent system uses a group of AI agents to complete large-scale and complex tasks with the increased compute power of a group.
“We see multiple agents collaborating, making decisions, sharing knowledge, and directing workflows. Sometimes under the supervision of an employee, and in future with increased levels of autonomy,” says Flower.
As the long march of technology continues towards automation, agentic AI may become just one inflection point along the way. But for now, companies must grapple with the risk reward conundrum and focus on implementation strategies to navigate a safe path.